Confronting the Risk of Shadow AI
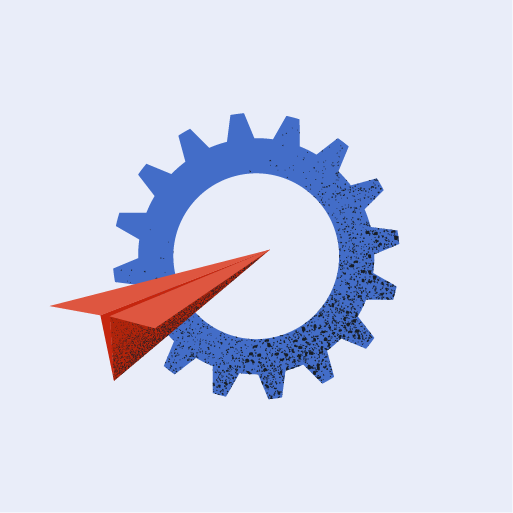
Close your eyes and try to picture the following scenario, which could be happening in your organization at this very moment: An employee uses a personal credit card to sign up for a software-as-a-service (SaaS) solution for generative artificial intelligence (GenAI). The solution touts its ability to create a customized large language model (LLM) based on your organization’s unique data set. It goes to work ingesting documents from your internal file repositories and quickly becomes capable of generating written content that’s aligned with your business needs. No one in your IT department, security team, or AI governance body has any idea that this is happening.
That’s good, right? Maybe not…. While it’s great that your employees are showing initiative, this kind of “shadow AI” activity is actually a serious source of risk exposure. Like shadow IT and shadow SaaS, shadow AI creates many different opportunities for security problems. These include data leakage, compliance violations, and legal liability. This article explores the nature of shadow AI and discusses way to mitigate the threat it poses.
What is Shadow AI?
Most well-run enterprises have established processes and policies for AI’s acquisition and deployment. Designated stakeholders in IT, security, compliance, and legal will typically weigh in on whether to adopt a particular AI tool, and if so, what safeguards need to be in place to prevent accidental data leaks and other problems. The term “shadow AI” refers to the use of AI that hasn’t gone through this vetting process. A shadow AI instance may function without anyone in authority knowing it exists.
Why does shadow AI happen? While it’s certainly possible that employees will act irresponsibility and ignore policy, it’s more likely that that shadow AI occurs by mistake. People may not be familiar with the policies. Or, and this is where real trouble can arise, they neither understand how AI works nor how the technology is embedded into the tools they are using.
Why Shadow AI is a Serious Source of Risk Exposure
To understand why shadow AI is problematic, it helps to have a grasp of the different types of AI software and how they work. A library full of books has been written on this topic, but briefly, AI is a field of computer science focused on developing software that can perform human-like reasoning, creativity, and task execution.
There are AI programs that analyze data and spot useful patterns, like social media sentiment. AI programs can examine texts, such as emails, and summarize them, e.g., Microsoft CoPilot. Robotic process automation (RPA) leverages AI to complete business process workflows. GenAI can write text and software code, create art, and so forth.
This varied set of use cases aside, all AI programs have one thing in common: They work by ingesting data and “training” to perform their designated work, e.g., reading millions of emails to learn how to spot fraud. The necessity to ingest data is one of the main drivers of security risk in AI, especially when AI software is running in the shadows.
Shadow AI is a source of risk exposure across multiple zones, including:
- Data breach/leakage, e.g., an AI program exfiltrating data to unauthorized parties.
- Compliance challenges, e.g., AI sharing customers’ private information, in violation of consumer privacy laws.
- Loss of intellectual property (IP), e.g., AI allowing unauthorized access to patent research or other IP.
- Disruption of operations, e.g., AI causing confusion among employees by generating incorrect information for use in business process workflows.
- Hallucinations, e.g., AI generating fictitious statements that might be confusing or offensive.
- Fraud, e.g., an RPA software robot programmed to steal customer credit card numbers.
Shadow AI vs. Shadow IT and Shadow SaaS
Shadow AI is comparable to, but different from, shadow IT and shadow SaaS. Shadow IT and shadow SaaS involve employees acquiring software (usually SaaS) or hardware, like mobile devices, without consulting the IT department or security team. Some of the security risks are similar, such as storing confidential corporate information on an easily accessible cloud platform like Google Docs or Box.com.
One difference is that shadow AI has the potential to reach into data repositories without anyone knowing what’s happening. An AI program can also execute tasks that are hidden from view. For these reasons, shadow AI’s reach and potential for damage is greater than that of shadow IT or shadow SaaS.
Another critical distinction is that shadow AI may occur inside of everyday applications that people are already using. It’s in the shadows, but also in broad daylight! The software industry expects to add AI features to its products in the coming years. As a result, employees might use AI without even knowing it’s there.
Shadow AI vs. Rogue AI
Shadow AI and rogue AI are comparable, and overlap, to a certain extent. However, they are different problems. Rogue AI comprises AI software that performs differently from the way it’s been instructed. This could be by accident, such as with a misconfiguration, but also due to hacking. A malicious actor who can gain access to AI source code or an administrative panel could instruct the software to commit fraud, harvest sensitive data, or generate written content that causes problems for a business.
Mitigating Shadow AI Risks
What can you do about shadow AI? A multi-threaded set of countermeasures is the best approach. No single security tool can identify and mitigate shadow AI. Basic cyber hygiene and well-informed employees are a given. To get to a strong security posture with shadow AI, it’s best to deploy the following combination of security solutions, configured for the anti-shadow AI use case:
- Data loss prevention (DLP) – which tracks data as it moves around and flags suspicious situations that indicate the presence of shadow AI.
- Cloud access security broker (CASB) – which detects and blocks shadow SaaS by monitoring and analyzing cloud user traffic.
- SaaS security data scanning – which identifies where SaaS apps have stored corporate data and flags suspicious instances that could be shadow AI.
- SaaS security user and session monitoring – which flags suspicious software activity that indicates shadow AI at work.
- SaaS security configuration monitoring, including third-party integration – which detects situations where a shadow AI application may be connecting with data sources that should be off limits.
Conclusion
Shadow AI is likely to be a widespread problem. It can occur by accident, with employees inadvertently allowing unauthorized AI software to tap into corporate data sources. Risks include data leakage, compliance violations, legal liability, and more. The trend of software companies embedding AI features into existing products will exacerbate the risk exposure. It is possible to mitigate the risk, however, using a multi-threaded approach that blends DLP, CASB, and different SaaS security methods, such as user and session monitoring. With this approach, it will be possible to reduce the potential impact of shadow AI on security, compliance, and operations.
Co-Founder & COO